Machine Learning of Semiempirical Parameters
We propose using machine learning (ML) for improving semiempirical Hamiltonian. Given sufficiently large training set ML can be used to correct parameters of semiempirical quantum chemical (SQC) method individually for any target molecule.
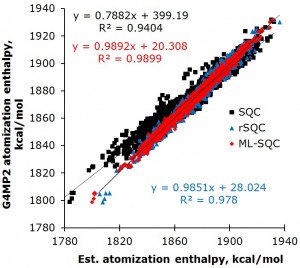
Such automatic parametrization technique (APT) stands in stark contrast to the traditional special-purpose reparametrization (SPR), when parameters are optimized for specific type of molecules and then resulting rSQC method is used unchanged for every other target molecule.
For our studies we used subset of huge database published by us. Hybrid ML-SQC approach has much lower error in predicted atomization enthalpies in comparison with SQC method with standard parameters.
The accuracy of ML-SQC technique is close to or even better than accuracy of many widely used DFT methods, while computational cost is by several orders of magnitude lower.
Brief overview of advantages (blue) and disadvantages (red) of APT is given below in comparison with traditional SPR approach.
APT | SPR |
Requires large training set | Training set can be relatively small |
Accuracy can be further improved by increasing training set | Accuracy is limited by the fixed functional form of the SQC model |
Approach consists of simple, computationally relatively cheap steps | Solving complex multidimensional optimization problem is necessary and is potentially computationally demanding |
Molecules far outside the training set are calculated with accuracy of the SQC method with standard parameters | Accuracy of the rSQC method is strongly deteriorated for molecules far outside the training set |
ML-SQC approach is well suited for big data applications, e.g. for fast and reasonably accurate high-throughput screening in drug or materials design.
Note that machine learning of parameters may be used not just for SQC methods, but also for other computational techniques that use parameters, e.g. in DFT or MD.
- Pavlo O. Dral, O. Anatole von Lilienfeld, Walter Thiel, Machine Learning of Parameters for Accurate Semiempirical Quantum Chemical Calculations. J. Chem. Theory Comput. 2015, Just Accepted Manuscript. DOI: 10.1021/acs.jctc.5b00141.
0 Comments on “Machine Learning of Semiempirical Parameters”